NIST: No Silver Bullet Against Adversarial Machine Learning Attacks
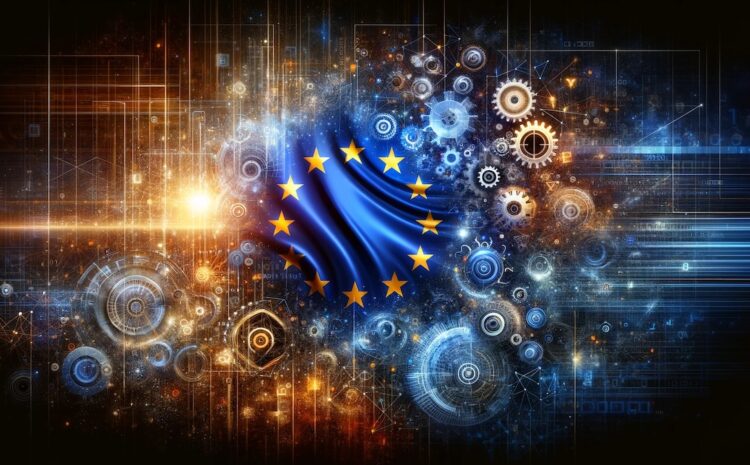
NIST has published a report on adversarial machine learning attacks and mitigations, and cautioned that there is no silver bullet for these types of threats.
Adversarial machine learning, or AML, involves extracting information about the characteristics and behavior of a machine learning system, and manipulating inputs in order to obtain a desired outcome.
NIST has published guidance documenting the various types of attacks that can be used to target artificial intelligence systems, warning AI developers and users that there is currently no foolproof method for protecting such systems. The agency has encouraged the community to attempt to find better defenses.
The report, titled ‘Adversarial Machine Learning: A Taxonomy and Terminology of Attacks and Mitigations’ (NIST.AI.100-2), covers both predictive and generative AI. The former focuses on creating new content, while the latter uses historical data to forecast future outcomes.
NIST’s report, authored in collaboration with representatives of Northeastern University and Robust Intelligence Inc, focuses on four main types of attacks: evasion, poisoning, privacy, and abuse.
In the case of evasion attacks, which involve altering an input to change the system’s response, NIST provides an attack on autonomous vehicles as an example, such as creating confusing lane markings that could cause a car to veer off the road.

In a poisoning attack, the attacker attempts to introduce corrupted data during the AI’s training. For example, getting a chatbot to use inappropriate language by planting numerous instances of such language into conversation records in an effort to get the AI to believe that it’s common parlance.
Attackers can also attempt to compromise legitimate training data sources in what NIST describes as abuse attacks.
In privacy attacks, threat actors attempt to obtain valuable data about the AI or its training data by asking the chatbot numerous questions and using the provided answers to reverse engineer the model and find weaknesses.
“Despite the significant progress AI and machine learning have made, these technologies are vulnerable to attacks that can cause spectacular failures with dire consequences,” said NIST computer scientist Apostol Vassilev. “There are theoretical problems with securing AI algorithms that simply haven’t been solved yet. If anyone says differently, they are selling snake oil.”
Joseph Thacker, principal AI engineer and security researcher at SaaS security firm AppOmni, commented on the new NIST report, describing it as “the best AI security publication” he has seen.
“What’s most noteworthy are the depth and coverage. It’s the most in-depth content about adversarial attacks on AI systems that I’ve encountered. It covers the different forms of prompt injection, elaborating and giving terminology for components that previously weren’t well-labeled. It even references prolific real-world examples like the DAN (Do Anything Now) jailbreak, and some amazing indirect prompt injection work,” Thacker said.
He added, “It includes multiple sections covering potential mitigations, but is clear about it not being a solved problem yet. It also covers the open vs closed model debate. There’s a helpful glossary at the end, which I personally plan to use as extra ‘context’ to large language models when writing or researching AI security. It will make sure the LLM and I are working with the same definitions specific to this subject domain.”
Troy Batterberry, CEO and founder of EchoMark, a company that protects sensitive information by embedding invisible forensic watermarks in documents and messages, also commented, “NIST’s adversarial ML report is a helpful tool for developers to better understand AI attacks. The taxonomy of attacks and suggested defenses underscores that there’s no one-size-fits-all solution against threats; however, understanding of how adversaries operate, and preparedness are critical keys to mitigating risk.”
“As a company that leverages AI and LLMs as part of our business, we understand and encourage this commitment to secure AI development, ensuring robust and trustworthy systems. Understanding and preparing for AI attacks is not just a technical issue but a strategic imperative necessary to maintain trust and integrity in increasingly AI-driven business solutions,” Batterberry added.